

What if we could predict the results for the incoming 2023 Rugby World Cup?

With the highly increasing number of data gathered with body sensors, statistics, and results about sports, it’s more and more conceivable to think about predicting (predict) sports’ related data. Some topics have been worth paying interest for several tech companies or independent programs such as ELO program, Lloyds Insurance, Turing Institute, and some less professional ones like Paul the Octopus.
But more than predicting the results, data science can give more hints about the sports itself: injuries prevention, sponsorships, tactics… The possibilities are (nearly) endless.
Data science is the ability to use numbers, texts, voice, videos, or any data to give a discovery, predictive, descriptive, or prescriptive analysis of them. Programmers use several programming languages to develop models able to simulate brain functions. Several mathematical-based functions such as regression or classification can predict results with machine learning models. For deep learning models, the technology of perceptrons (simulation of a neuron) is used to learn and adapt as a human brain would do. For more info about machine learning and deep learning, you can read this article (Difference Between Machine Learning and Deep Learning, Geeksforgeeks, 07 Feb 2023).

To develop a reliable and precise model, you need to focus on:
- Quality: use of the body sensors data, precise metrics, powerful algorithms, and reliable/fast storage. Despite the good formatting and quality, the data must be relevant and linked to the subject to treat.
- Quantity: time-based data, field data, players data. Every data should be in a sufficient amount to give enough hints to the model to learn but not too much to not overtrain it.
Applications of AI in the sports industry are twofold. On the one hand, sports clubs and sports teams benefit from better decision-making concerning on and off-pitch performances. On the other hand, it also benefits other sport industry stakeholders like sport betting companies to increase revenue.
Below, we will explain in more detail how Data Science can improve decision-making in sports with some examples.
Scouting
With the example of the book/movie Moneyball, data is an opportunity for teams to detect and discover the most relevant players for their position, qualities, and for the team itself. Moreover, based on all the parameters decided (budget, role, weight, main hand, …), the scouting possibilities leverage the capacity of each team to gather multiple talented members.
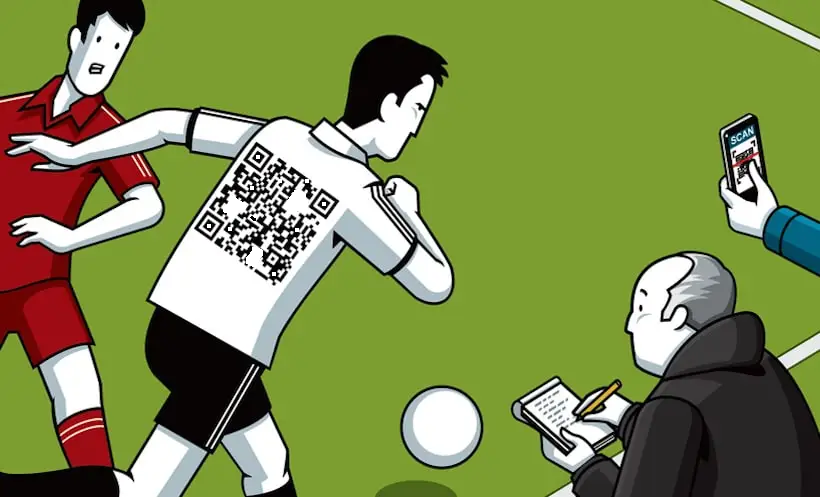
Athlete’s physical abilities

One objective for leveraging sensor data into sports is to ensure that athletes are at their best performance at the right moment. Hence, it is possible to tailor suit pieces of training but also use these sensors to prevent injuries and to minimize the downtime of athletes. These body sensors such as heart rate, distance covered, running speed, acceleration, jumps and turns, players’ sprint, position in the field, and relative to other players coercively with their impacts help build better models and prevent injuries.
One example of the data sensors’ reliance on sport is the USA women’s cycling team that partnered with IBM enabling the team to get insights and better performance during races. After a year of collaboration, the team won the Silver medal in the Olympics in Rio.
Tactic
Using data in sports is not only targeted for medical purposes but also for analyzing the performance of tactics. In the case of the NFL, player data is shared league-wide to enable clubs to analyze them. Though there are no figures proving data efficiency regarding on-track performances. The gap widens between an early adopter and a laggard in the adoption of the technology.
Sponsorships
Data Science in sports offers the possibility to increase sponsors’ visibility. For example, during the broadcast of sport on television, data can be collected to analyze camera coverage of a football game, formula 1 race to optimize sponsors’ visibility.
Sports betting
This steady reliance on data in sports is also a revolution taking place in sport betting companies. For example, to increase revenue, these data can be used to predict and propose more accurate odds. Consequently, they can also offer a wider variety of odds proposed. In a larger scope, sports betting is also transformed by greater reliance on data regarding the strategy of targeted marketing and analyzing customer behavior.

https://www.theringer.com/platform/amp/nfl/2018/12/19/18148153/nfl-analytics-revolution
https://www.dailydot.com/debug/usa-cycling-womens-pursuit-ibm-watson-sensors/
https://www.geeksforgeeks.org/difference-between-machine-learning-and-deep-learning/
https://sportsmedicine-open.springeropen.com/articles/10.1186/s40798-021-00372-0